1. Introduction:
Farming has always been at the mercy of nature, unpredictable weather, and manual labor. Despite advancements in agricultural techniques, many farmers still struggle to maintain efficiency from the soil to harvest. As global demand for food continues to rise, the agriculture sector faces significant challenges, including labor shortages, soil degradation, and climate change. This reality raises a crucial question: How can modern farmers enhance efficiency and optimize crop yields without depleting resources?
Enter Artificial Intelligence (AI) – a transformative technology reshaping agriculture from the ground up. By leveraging AI-driven solutions, farmers can make data-backed decisions that improve productivity, reduce waste, and ensure sustainable farming practices. From precision farming to smart irrigation systems, AI applications are becoming more accessible and practical, even for small-scale farmers. This transformation is not just about integrating high-tech gadgets but fundamentally rethinking traditional farming practices.
One of the most exciting developments is the use of predictive analytics to monitor crop health and anticipate potential issues. Imagine being able to predict a pest outbreak before it happens or optimize irrigation based on real-time soil data. Such innovations not only save valuable resources but also maximize yields, directly impacting the farmer’s bottom line. Additionally, autonomous machines are revolutionizing the way farmers manage labor-intensive tasks like planting, weeding, and harvesting.
However, implementing AI in agriculture is not without challenges. High initial costs, lack of technical expertise, and data privacy concerns can hinder adoption, especially in regions where farming practices are still predominantly manual. Addressing these challenges requires collaboration between tech developers, policymakers, and farming communities to ensure that AI technologies are both accessible and scalable.
In this article, we will explore the critical role AI plays in modern agriculture, examining how it enhances efficiency from soil analysis to harvest optimization. We will also look at real-world applications, discuss potential barriers, and consider the future of AI-driven farming. Whether you are a tech enthusiast or a farmer looking to modernize operations, this guide offers practical insights into the power of AI in agriculture.
2. Identifying the Problem: Efficiency Gaps in Modern Farming
Despite the rapid advancements in agricultural technology, many farmers around the world continue to face significant challenges in maintaining efficiency throughout the crop production cycle. Traditional farming practices, while time-tested, often fall short in addressing modern agricultural demands. Issues like resource overuse, labor inefficiency, and unpredictable environmental factors can significantly hinder productivity. These gaps create a pressing need for innovative solutions that can seamlessly integrate with existing farming methods.
The Problem with Traditional Irrigation:
One of the most prominent inefficiencies lies in irrigation practices. Many farms still rely on manual watering or outdated irrigation systems, leading to water wastage and inconsistent soil moisture levels. In regions where water scarcity is already a concern, inefficient irrigation not only reduces crop yields but also puts additional pressure on local water resources. For instance, farms that do not use smart irrigation systems often end up overwatering or underwatering crops, compromising plant health and growth.
Labor-Intensive Crop Monitoring:
Monitoring crop health manually is another area where traditional practices falter. Farmers often need to inspect large fields by hand, which is time-consuming and prone to human error. Moreover, identifying pests or nutrient deficiencies at an early stage can be incredibly challenging without the aid of modern technology. As a result, issues like pest infestations or nutrient imbalances often go unnoticed until significant damage has occurred. In contrast, AI-driven crop monitoring systems can detect anomalies through machine learning algorithms, providing early warnings that allow for timely intervention.
Harvest Inefficiencies:
Manual harvesting, while labor-intensive, remains the norm in many farming communities. This approach not only slows down the harvesting process but also increases the risk of produce loss due to delays. In contrast, autonomous harvesting robots can perform tasks more efficiently, reducing waste and ensuring that crops are picked at optimal ripeness. This technology significantly improves yield quality and reduces post-harvest loss.
Why Addressing These Gaps Matters:
Efficiency gaps in modern farming are not just minor inconveniences, they directly impact the economic viability of agricultural operations. Addressing these challenges requires adopting AI-driven solutions that offer precision farming capabilities, including automated monitoring, irrigation, and harvesting. By modernizing these critical stages, farmers can achieve sustainable production while minimizing resource use and labor demands.
In the following sections, we will explore how AI technologies can bridge these efficiency gaps, making farming more productive, sustainable, and economically viable.
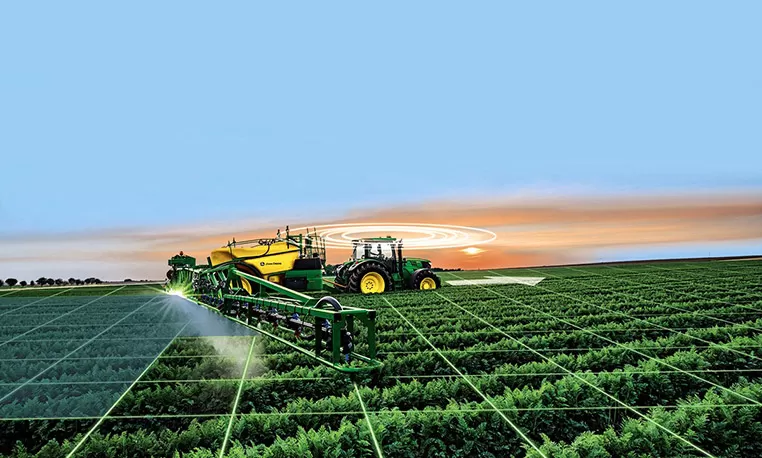
3. AI-Driven Solutions for Enhanced Efficiency
To address the pressing efficiency gaps in modern agriculture, farmers are increasingly turning to artificial intelligence (AI) for solutions that streamline processes, optimize resource usage, and increase yields. By integrating AI at every stage, from soil preparation to harvesting, farmers can move away from traditional methods that are often labor-intensive and prone to error. Instead, they can embrace data-driven, automated solutions that significantly enhance productivity.
3.1 Precision Soil Management with AI:
One of the key applications of AI in agriculture is in soil health management. AI-powered sensors and data analytics allow farmers to monitor soil conditions in real time, including moisture levels, pH balance, and nutrient content. These insights help in making precise decisions about fertilizer application and irrigation schedules, preventing both overuse and underuse of resources. For example, predictive soil management systems can analyze historical and current data to recommend optimal planting times and fertilizer dosages.
3.2 Smart Irrigation Systems:
Water management is crucial in farming, and AI-driven smart irrigation systems are making a significant impact. These systems use data from soil moisture sensors and weather forecasts to regulate watering schedules automatically. For instance, AI-based irrigation can determine the exact amount of water needed for each crop, reducing wastage and promoting sustainable water usage. In areas where water scarcity is a major issue, adopting these systems has proven to improve crop resilience and yield.
3.3 AI in Crop Health Monitoring:
Monitoring plant health manually across vast fields is impractical, especially during critical growth stages. With AI-driven drone surveillance and machine learning algorithms, farmers can detect diseases, pests, and nutrient deficiencies early on. These systems use image recognition technology to scan crops for visual signs of stress, such as leaf discoloration or spotting. Once detected, automated alerts prompt farmers to take immediate action, minimizing crop loss and maintaining yield quality.
3.4 Autonomous Harvesting:
Labor shortages and the high cost of manual harvesting present significant challenges, especially for large-scale farms. To overcome this, farmers are adopting autonomous harvesting robots that can pick, sort, and package crops efficiently. These robots use computer vision to identify ripe produce, ensuring that only mature crops are harvested. As a result, post-harvest losses decrease, and labor costs are significantly reduced.
3.5 Real-Time Decision Support Systems:
Modern farms generate vast amounts of data, but without actionable insights, data alone is not enough. AI-driven decision support systems analyze data from various sources, such as soil sensors, weather reports, and crop growth data, to offer real-time recommendations. Whether it’s optimizing irrigation schedules or planning the harvest, these systems help farmers make informed choices that improve efficiency and profitability.
4. Case Study: Boosting Yields with AI Precision Farming
To truly understand the transformative impact of AI on agriculture, it’s essential to look at real-world applications. Across the globe, farmers are leveraging AI-driven precision farming techniques to enhance crop yields and optimize resource use. In this section, we will explore two noteworthy case studies that demonstrate how AI is making a tangible difference in farming efficiency.
1. California Vineyard: Smart Irrigation and Yield Optimization
One prominent example comes from a vineyard in California, where water scarcity poses a constant challenge. The vineyard adopted an AI-based smart irrigation system designed to optimize water usage without compromising vine health. By using soil moisture sensors integrated with predictive algorithms, the system could precisely gauge when and how much to water.
The AI-driven approach allowed the vineyard to reduce water consumption by 30% while maintaining grape quality and yield. Furthermore, integrating drones with multispectral cameras provided data on vine health, detecting early signs of drought stress or disease. The system’s ability to monitor large fields efficiently saved time and reduced labor costs, leading to increased profitability.
Key Takeaways:
- Reduced water usage by 30%
- Improved crop health monitoring through drone surveillance
- Enhanced yield quality and reduced operational costs
2. Indian Rice Farm: Predictive Yield Forecasting
In India, a large rice farming cooperative faced challenges in predicting yield outcomes due to unpredictable weather patterns. By adopting AI-powered predictive analytics, the cooperative could analyze historical climate data, soil conditions, and crop performance metrics to forecast potential yields accurately.
The system’s advanced algorithms identified patterns correlating to higher yields, such as optimal planting dates and soil nutrient levels. As a result, farmers were able to adjust their planting strategies accordingly. In one season, the cooperative reported a 15% increase in yield compared to previous years, directly attributed to better planning and precise fertilizer management.
Key Takeaways:
- Increased yield by 15%
- Data-driven decisions on planting and fertilization
- Minimized crop losses due to better risk prediction
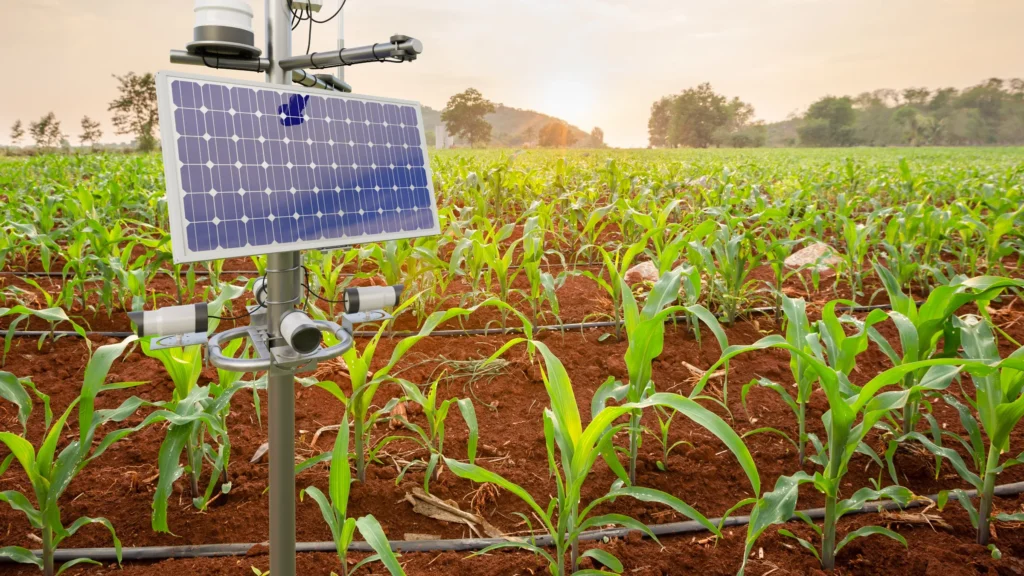
5. Addressing the Concerns: Cost, Accessibility, and Ethics
While the potential of AI-driven farming is undeniably promising, several challenges hinder its widespread adoption. Despite the impressive outcomes seen in case studies, many farmers, particularly those in developing regions, face barriers related to cost, accessibility, and ethical considerations. Understanding these challenges is essential to promoting AI’s responsible and sustainable use in agriculture.
1. High Initial Investment:
One of the primary obstacles is the high upfront cost associated with AI technologies. Setting up systems like smart irrigation, autonomous tractors, or drone-based crop monitoring requires significant capital. For small and medium-sized farms, the financial burden can be overwhelming. Even after installation, maintenance, and software updates add ongoing expenses.
2. Lack of Technical Expertise:
Operating AI-driven systems often demands a certain level of technical knowledge. For farmers accustomed to traditional methods, adopting AI can seem daunting. In rural areas with limited internet access or inadequate training resources, the learning curve becomes even steeper. To mitigate this, there is a need for training programs tailored to farmers, focusing on practical usage rather than technical jargon.
3. Data Ownership and Privacy Issues:
As AI systems collect vast amounts of data on soil health, crop conditions, and yield predictions, questions about data ownership and privacy arise. Farmers may feel uncomfortable sharing sensitive farm data with third-party companies, especially when it is unclear how the data will be stored, used, or shared.
4. Ethical Concerns:
The ethical implications of replacing human labor with autonomous systems also deserve attention. In areas where agriculture is the primary source of employment, automation could displace workers, leading to social and economic challenges. Balancing automation with human employment requires thoughtful integration strategies, such as focusing on collaborative robots (cobots) that enhance human capabilities rather than replacing them.
6. Conclusion: Embracing the Future of AI-Driven Farming
The journey from soil to harvest has always been a complex and demanding process for farmers. As the agricultural sector grapples with challenges like resource scarcity, labor shortages, and climate unpredictability, the integration of Artificial Intelligence (AI) offers a promising way forward. Throughout this article, we have explored how AI-driven solutions are transforming farming efficiency, from precision soil management to autonomous harvesting.
A Paradigm Shift in Agriculture:
AI is not just an add-on to traditional farming practices; it represents a fundamental shift in how agriculture is managed and optimized. The real-world examples we discussed, like the Californian vineyard’s smart irrigation system and the Indian rice farm’s predictive yield forecasting, demonstrate how data-driven decisions lead to better resource utilization and higher yields. These case studies highlight the potential for AI to increase productivity while also promoting sustainable practices.
However, adopting AI in agriculture is not without its challenges. High initial costs, lack of technical expertise, and concerns over data ownership can make the transition daunting, especially for small-scale farmers.
The Road Ahead:
Looking to the future, the key to successful AI adoption lies in building solutions that are cost-effective, user-friendly, and ethically sound. Farmers should not feel overwhelmed by complex technology; instead, AI tools should be designed to complement their existing practices. Moreover, fostering partnerships between agritech companies, research institutions, and local farming cooperatives will be essential for spreading awareness and ensuring practical implementation.
A Call to Action:
Farmers and agribusiness stakeholders should take proactive steps in exploring available AI technologies suited to their unique needs. Whether it’s adopting smart irrigation systems or leveraging crop health monitoring tools, even small advancements can make a significant difference. The future of agriculture lies in embracing innovation, and AI is at the forefront of that transformation.
In closing, the path from soil to harvest will always involve hard work and dedication, but with AI’s assistance, it becomes a journey marked by efficiency, sustainability, and growth. Stay informed, stay innovative, and lead the way toward smarter farming practices.
FAQs:
Q1. How is AI improving farming efficiency?
AI is enhancing farming efficiency by automating tasks such as crop monitoring, irrigation management, and harvesting. Using machine learning algorithms, AI helps farmers make data-driven decisions, reduce resource wastage, and improve yield quality by predicting weather patterns, detecting pests, and optimizing water usage.
Q2. What are the main AI technologies used in modern farming?
The primary AI technologies used in agriculture include precision farming, smart irrigation systems, drone-based crop monitoring, and autonomous harvesting robots. These tools allow for real-time analysis of soil conditions, crop health, and environmental factors, making farming practices more efficient and sustainable.
Q3. Can AI really help small-scale farmers?
Yes, AI can benefit small-scale farmers by providing access to affordable technology solutions that optimize resources. Through mobile apps and affordable AI tools, small farms can use AI-driven crop monitoring and predictive analytics to improve productivity and reduce costs. Additionally, subsidies or partnerships can help reduce the initial investment barrier.
Q4. What challenges do farmers face when adopting AI technology?
Farmers face several challenges when adopting AI, including high initial costs, lack of technical expertise, and concerns over data privacy. Overcoming these hurdles requires support in the form of training programs, financial assistance, and clear data ownership policies to build trust in AI solutions.
Q5. How does AI help with sustainability in farming?
AI promotes sustainability by reducing resource waste, such as water and fertilizer. Smart irrigation systems ensure that crops receive the right amount of water, while AI-driven monitoring helps identify plant diseases early, reducing the need for harmful chemicals. Overall, AI enables more environmentally-friendly and efficient farming practices.
Leave a comment