1. Introduction:
In a world where feeding over 9 billion people by 2050 is a looming challenge, the agricultural sector is under immense pressure to evolve. Traditional farming methods, while foundational, often fall short in tackling modern issues like climate unpredictability, resource scarcity, and rising operational costs. Enter smart farming, a revolutionary approach that leverages artificial intelligence (AI) to optimize every aspect of agricultural production.
Smart farming, also referred to as precision agriculture, uses technologies such as machine learning, computer vision, and IoT sensors to make farming more efficient, data-driven, and sustainable. It enables farmers to predict crop yields, monitor soil health in real time, automate irrigation, and even detect pests before they cause significant damage. As a result, AI in agriculture isn’t just a futuristic concept, it’s a present-day necessity.
The real problem lies in the widening gap between food demand and agricultural productivity. Farmers need intelligent tools that go beyond manual labor or intuition. With smart farming systems, data becomes a powerful ally, transforming reactive farming into predictive farming. This shift is especially vital for smallholders who face limited resources and climate challenges.
In this article, we’ll explore how AI-powered solutions are reshaping agriculture, from farm management systems to autonomous machinery. We’ll also look at real-world use cases and address the ethical and logistical concerns of AI adoption.
The era of smart farming isn’t on the horizon, it’s already here. The question is: are we ready to fully embrace it?
2. What Is Smart Farming?
Smart farming is the integration of cutting-edge technologies like artificial intelligence, IoT, robotics, and big data into traditional agricultural practices. Its primary goal is to enhance decision-making and automate routine processes, leading to more productive, sustainable, and precise farming operations.
Unlike conventional methods that rely heavily on manual labor and intuition, smart farming employs data-driven systems to analyze everything from soil moisture to crop health and weather forecasts. These systems allow farmers to make real-time, informed decisions about irrigation, fertilization, pest control, and harvesting, reducing waste and maximizing output.
One of the core concepts within smart farming is precision agriculture. This technique focuses on observing, measuring, and responding to inter- and intra-field variability in crops. For example, drones and satellite imagery help map farmland, detect crop stress early, and monitor plant growth stages with pinpoint accuracy.
Another pillar of smart farming is farm management software, which consolidates data from various sources and provides actionable insights. With predictive analytics powered by machine learning algorithms, these platforms can estimate yield outcomes, optimize resource usage, and forecast potential issues, often before they become visible to the naked eye.
The result is a new generation of AI-driven farming systems that not only boost productivity but also support sustainable agriculture by minimizing environmental impact.
In essence, smart farming marks the transformation of agriculture from a manual, reactive practice into a technology-enabled, proactive system, helping both large agribusinesses and smallholder farmers compete in a rapidly changing world.
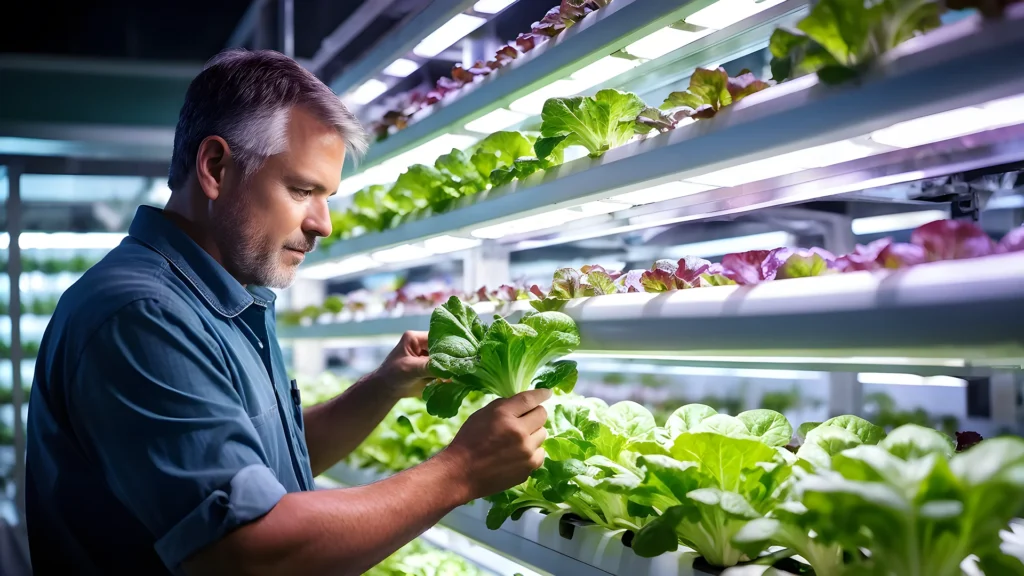
3. How AI Is Powering Smart Farming
Artificial Intelligence (AI) is the driving force behind smart farming, transforming raw agricultural data into meaningful, actionable insights. By analyzing vast amounts of information from IoT sensors, satellite imagery, drones, and weather forecasts, AI empowers farmers to make smarter, faster decisions that improve efficiency and crop health.
One of the most impactful applications of AI in agriculture is crop monitoring. Using computer vision and machine learning, AI models can detect diseases, pest infestations, and nutrient deficiencies early, often before they become visible to the human eye. This enables farmers to act quickly and prevent large-scale damage, reducing reliance on pesticides and minimizing crop loss.
Another critical area is predictive analytics. AI-powered platforms analyze historical yield data, weather trends, and soil conditions to forecast future outcomes with impressive accuracy. These insights help farmers plan sowing schedules, irrigation cycles, and harvest times, leading to optimized output and reduced waste.
AI-based irrigation systems are also becoming common in smart farms. These systems use real-time data to determine exactly when and how much water each part of a field needs, conserving water and ensuring optimal crop hydration.
Furthermore, AI enables autonomous machinery like smart tractors and robotic harvesters to perform complex tasks without human intervention, improving efficiency and reducing labor costs.
AI doesn’t just automate agriculture, it enhances decision-making, improves yield prediction, and supports long-term sustainability goals.
4. Benefits of AI in Agriculture
The integration of AI into farming practices offers numerous tangible benefits that extend beyond just higher yields. At its core, AI in agriculture enhances precision, efficiency, and sustainability, allowing farmers to do more with less.
One of the most significant advantages is resource optimization. With AI-based systems monitoring soil health, weather patterns, and crop conditions, farmers can apply water, fertilizers, and pesticides exactly where and when needed. This not only reduces costs but also minimizes environmental impact, a win for both the farmer and the planet.
AI also contributes to yield prediction accuracy. By analyzing years of data on climate, soil, and crop performance, machine learning models can forecast production outcomes, helping farmers make proactive decisions about planting and harvesting. This reduces risk, improves planning, and increases profitability.
Labor efficiency is another key benefit. With the rise of agricultural robotics and smart machinery, time-consuming tasks like weeding, spraying, or harvesting can be automated. This is particularly helpful in regions facing agricultural labor shortages.
Moreover, AI systems provide real-time alerts for pest infestations, disease outbreaks, or weather anomalies, allowing for faster response times and reduced crop losses.
Perhaps most importantly, AI enables data-driven decision-making, empowering even small-scale farmers with insights that were once only available to large agribusinesses.
Ultimately, AI makes farming more predictable, scalable, and resilient, qualities that are essential in today’s unpredictable climate and market landscape.
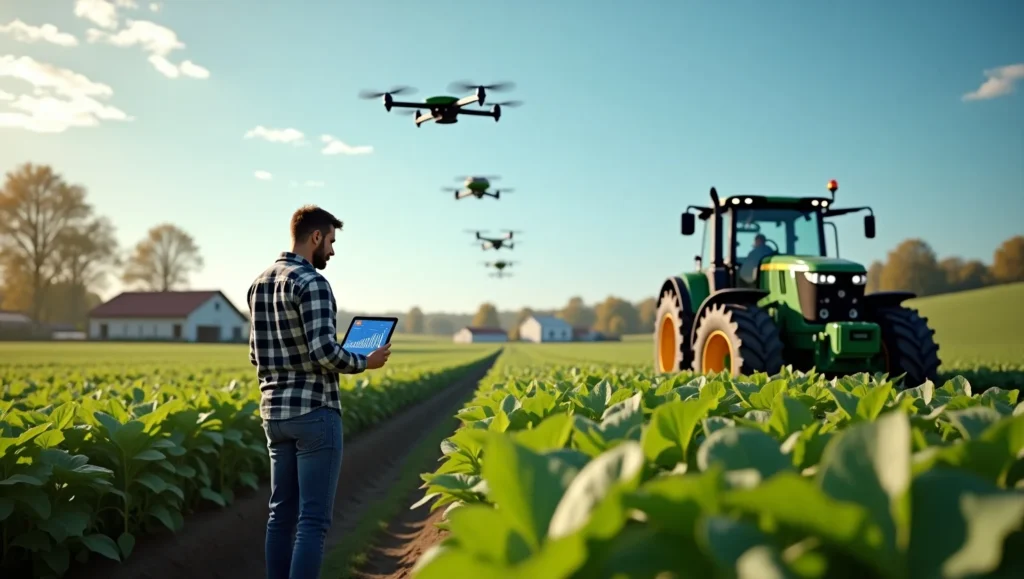
5. Real-World Examples of AI in Action
The promise of AI in agriculture isn’t theoretical, it’s already being realized across farms worldwide. From major agritech companies to smallholder farmers, real-world applications of AI-powered smart farming are demonstrating tangible results.
Take John Deere, for instance. The company has developed autonomous tractors equipped with AI and computer vision that can plow, plant, and spray crops with pinpoint accuracy. These machines reduce the need for manual labor and ensure uniform coverage, which boosts productivity while minimizing waste.
In India, Microsoft’s AI Sowing App has helped small-scale farmers increase yields by up to 30%. By analyzing historical climate data, weather forecasts, and soil health metrics, the app recommends optimal sowing dates. It has made a significant impact in regions where traditional farming decisions were based on intuition.
Another example is Prospera, an AI startup that offers real-time crop analytics using camera-equipped sensors. Their technology helps greenhouse farmers detect issues early, from irrigation stress to pest problems, improving both yield and quality.
Even drone technology, powered by AI and computer vision, is transforming large-scale farming. Companies like PrecisionHawk use drones to map fields, assess plant health, and monitor crop development, enabling faster and more accurate interventions.
These case studies show that AI is more than a tool; it’s a strategic partner in modern farming. Whether through robotics, mobile apps, or analytics platforms, AI is helping farmers around the world grow smarter, not just more.
6. Challenges and Ethical Considerations
While the benefits of AI in agriculture are undeniable, its widespread adoption is not without challenges. From technological limitations to ethical dilemmas, the path to smart farming requires careful navigation.
One of the biggest concerns is the digital divide. Many smallholder farmers, especially in developing countries, lack access to reliable internet, modern equipment, or even basic digital literacy. This limits their ability to adopt AI tools, potentially widening the gap between tech-enabled agribusinesses and traditional farms.
Data privacy and ownership are other pressing issues. AI systems collect vast amounts of data, from soil conditions to crop patterns, but who owns this data? If managed by private companies, farmers risk losing control over valuable information that should benefit their operations, not external stakeholders.
There’s also the fear of job displacement. As agricultural automation increases, concerns grow around the reduction of manual labor jobs in rural communities. While AI can ease labor shortages, it must be introduced in a way that supports economic inclusion, such as upskilling workers for tech-based roles.
Ethical considerations around algorithmic bias also come into play. If AI models are trained on incomplete or skewed datasets, the recommendations could be inaccurate or harmful, especially in diverse farming contexts with varying climates, crops, and socioeconomic factors.
Lastly, high initial costs for AI technology can be a barrier. Without subsidies or public-private partnerships, many farmers simply can’t afford to implement these systems.
To truly transform agriculture, we must not only innovate but also do so responsibly.
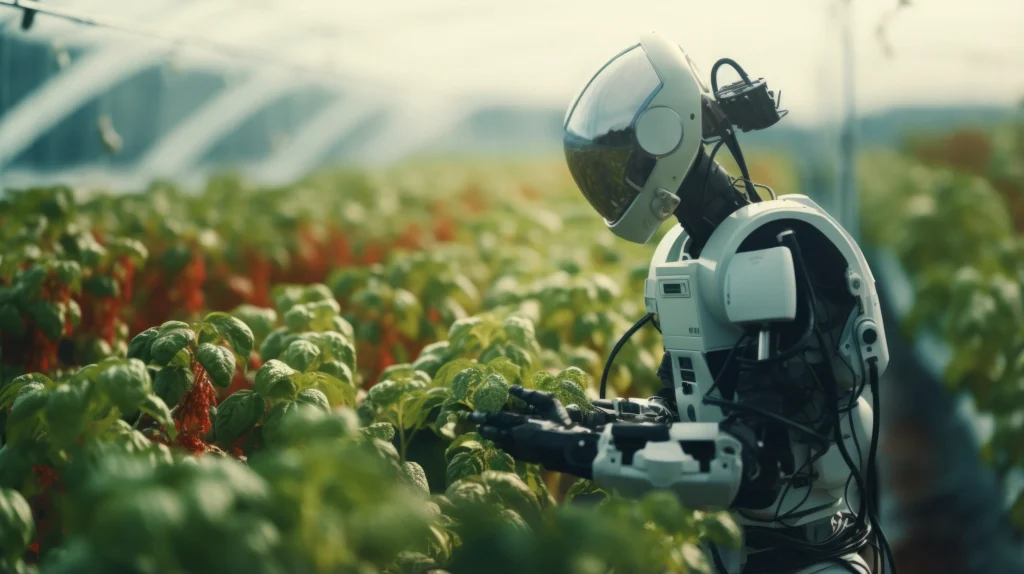
7. Conclusion and Future Outlook
As we’ve seen, AI in agriculture is reshaping the industry, offering innovative solutions that improve productivity, sustainability, and efficiency. From smart tractors to AI-powered crop monitoring, the potential of AI to enhance farming practices is immense. It’s clear that smart farming is not just a trend; it’s the future of agriculture.
However, the widespread adoption of AI in farming depends on overcoming key challenges, such as addressing the digital divide, ensuring data privacy, and mitigating job displacement. The agricultural community must work together to create a more inclusive environment where both large and small farms can benefit from these technological advancements.
The future of AI in agriculture looks promising. As machine learning algorithms continue to evolve, the accuracy of predictions and the efficiency of autonomous systems will only improve. In addition, the expansion of 5G networks and the Internet of Things (IoT) will make it easier for farmers to collect and analyze real-time data from every corner of their fields.
Moreover, AI’s potential to improve food security cannot be overstated. By optimizing resource use and minimizing waste, AI can help ensure that the agricultural sector is better equipped to meet the growing global food demand. As the industry embraces precision agriculture and more sustainable farming methods, AI will be a critical tool in tackling challenges such as climate change and resource scarcity.
In conclusion, AI is no longer just a tool for the future; it’s a reality shaping the present and paving the way for a more sustainable and efficient agricultural landscape. The question remains: how will we harness this technology to benefit everyone?
FAQs:
Q1. What is smart farming, and how does AI enhance it?
Smart farming integrates digital technologies like AI, IoT, and robotics into agricultural practices to improve efficiency and sustainability. AI enhances smart farming by analyzing data from various sources, such as soil sensors, weather stations, and drones, to make informed decisions on irrigation, fertilization, and pest control. This leads to optimized resource use, increased yields, and reduced environmental impact.
Q2. How does AI improve crop yield prediction and resource management?
AI utilizes machine learning algorithms to analyze historical data, weather patterns, and soil conditions, enabling accurate predictions of crop yields. This information helps farmers plan planting and harvesting schedules effectively. Additionally, AI-driven systems optimize resource management by determining the precise amount of water, fertilizers, and pesticides needed, minimizing waste and promoting sustainable farming practices.
Q3. What are the benefits of using AI in agriculture?
The adoption of AI in agriculture offers several benefits:
– Increased Efficiency: Automation of tasks like planting, watering, and harvesting reduces labor costs and time.
– Enhanced Precision: AI systems provide accurate data analysis, leading to better decision-making in crop management.
– Sustainability: Optimized use of resources reduces environmental impact and promotes eco-friendly farming practices.
– Cost Savings: Efficient resource management and reduced waste translate to lower operational costs.
Q4. What challenges do farmers face when implementing AI technologies?
Farmers encounter several challenges when adopting AI technologies:
– High Initial Costs: The investment required for AI tools and infrastructure can be substantial.
– Digital Divide: Limited access to high-speed internet and technology in rural areas hinders AI adoption.
– Data Privacy Concerns: Farmers may be wary of sharing sensitive data with third-party AI service providers.
– Technical Expertise: A lack of skilled personnel to operate and maintain AI systems can impede implementation.
Q5. How is AI being used to combat climate change in agriculture?
AI assists in combating climate change by:
– Predicting Weather Patterns: AI models forecast weather conditions, allowing farmers to prepare for adverse events like droughts or floods.
– Optimizing Resource Use: AI systems ensure efficient use of water and fertilizers, reducing waste and conserving resources.
– Monitoring Environmental Conditions: AI-driven sensors detect changes in soil health and crop conditions, enabling timely interventions to mitigate environmental stress.
Leave a comment