1. Introduction:
Artificial Intelligence (AI) is transforming nearly every industry, and agriculture is no exception. From drones monitoring crop health to algorithms predicting weather patterns, AI in farming is rapidly gaining traction. With promises of improved yields, reduced waste, and smarter resource use, it’s easy to see why precision agriculture has become a major talking point among agri-tech enthusiasts. But behind this optimism lies a complex web of ethical and economic challenges that demand attention.
As AI adoption in agriculture grows, so do concerns about data privacy, farmer autonomy, and the growing digital divide between large agribusinesses and smallholder farmers. Many of these challenges are not just technical, they’re deeply human. Who owns the data collected by autonomous tractors? Will AI-driven automation displace labor or simply shift it? Are AI systems, often trained on biased or incomplete data, making fair decisions?
These questions aren’t just academic, they impact real lives, especially those of rural communities and family farms. Without clear regulatory frameworks and inclusive design, there’s a risk that AI tools may benefit only a few while sidelining the rest. The ethical challenges of AI in agriculture go hand-in-hand with economic risks, including high upfront costs and uncertain returns on investment.
This article dives deep into these pressing concerns, exploring both the opportunities and the unintended consequences of AI in farming. As we face a future shaped by technology, it’s time to ask: Is AI truly helping farmers, or are we sowing the seeds of inequality?
2. AI in Farming: Benefits and Limitations
The use of AI in farming is revolutionizing how crops are grown, monitored, and harvested. By integrating machine learning algorithms, drones, and Internet of Things (IoT) sensors, farmers can now make real-time, data-driven decisions. These tools offer enhanced precision in irrigation, fertilization, and pest control, leading to increased yields and reduced environmental impact. This form of precision agriculture is one of the most promising applications of AI in agriculture.
For instance, AI-powered satellite imagery and sensor data can detect signs of crop stress or disease before they become visible to the naked eye. Similarly, autonomous tractors and smart irrigation systems help conserve water and labor, two of the most constrained resources in modern farming. These innovations align with the growing need for sustainable and scalable food production systems.
However, despite these advantages, the limitations of AI in agriculture cannot be ignored. One major hurdle is its low adoption rate, especially in labor-intensive and small-scale farming. According to recent studies, the agriculture sector shows minimal AI penetration, with only 0.1% of AI applications deployed here. High setup costs, lack of digital literacy, and inadequate infrastructure further limit its reach.
Additionally, AI systems can sometimes provide misleading recommendations due to biased or incomplete datasets. Over-reliance on algorithmic decisions may lead to ecological trade-offs or poor field outcomes. As we embrace smart farming technologies, it’s crucial to balance innovation with caution, ensuring that AI solutions are accessible, transparent, and truly beneficial across all scales of farming.
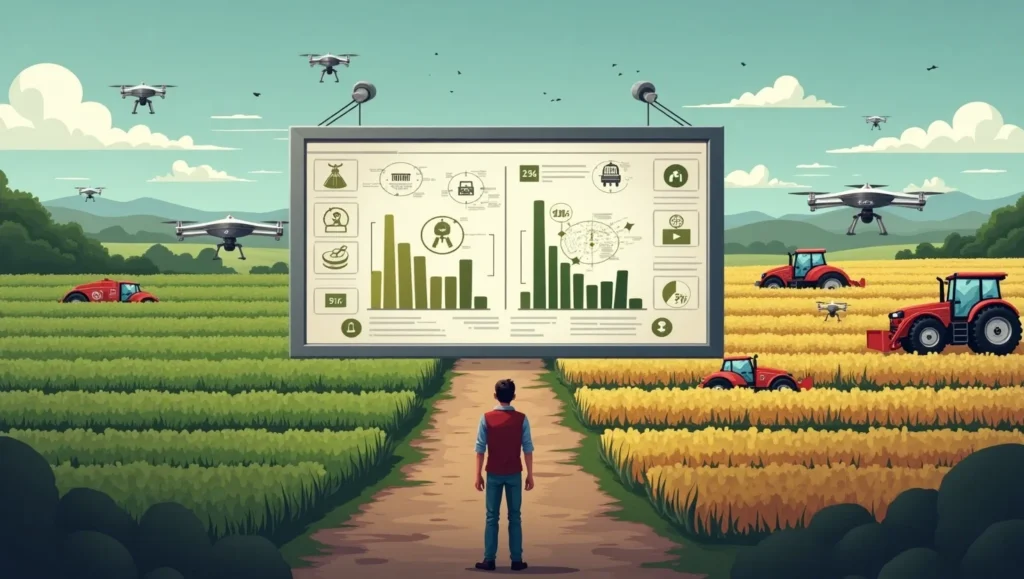
3. Ethical Challenges: Data, Privacy, and Fairness
As AI tools become more embedded in modern agriculture, they raise serious ethical questions about who controls the data, how decisions are made, and whether these systems serve all farmers equally. One of the most pressing issues is data ownership. Precision agriculture relies heavily on real-time data from soil sensors, drones, and machinery. However, in many cases, farmers don’t truly own this information; agri-tech companies often store and analyze it under vague or one-sided contracts, limiting farmers’ control over their own farm data.
This lack of clear data governance poses privacy risks and creates power imbalances. When tech providers dictate the terms of use, farmers may unknowingly give up rights to sensitive information. These practices mirror concerns raised in other sectors, where algorithmic transparency is lacking. AI in farming often functions as a “black box,” generating recommendations or decisions without clearly explaining the rationale behind them.
Furthermore, fairness becomes a growing concern when AI tools are trained on biased datasets that favor large-scale operations or specific regions. As a result, smallholder farmers may receive less accurate recommendations or be excluded altogether from AI-driven platforms. Insurance models powered by AI can also amplify inequality, charging higher premiums based on risk assessments drawn from partial or skewed data.
These ethical challenges underscore the urgent need for transparent, accountable, and equitable AI systems in agriculture. Without proper oversight, there’s a real danger that AI adoption could deepen existing inequalities rather than bridge them, putting the future of small farms at even greater risk.
4. Economic Impacts: Costs, Adoption Barriers, and Inequality
While AI promises increased efficiency in agriculture, its economic implications reveal a mixed picture, especially for small and medium-scale farmers. High implementation costs are a major barrier to entry. AI-powered solutions such as autonomous machinery, satellite mapping tools, and data analytics platforms often come with steep price tags, making them accessible primarily to well-capitalized agribusinesses. This raises a critical question: Is AI in agriculture financially viable for all farmers?
The return on investment (ROI) in AI tools remains uncertain for many growers. Factors like unpredictable weather, fluctuating commodity prices, and limited access to digital infrastructure can hinder the effective use of smart farming technologies. For smallholders in developing regions, even basic connectivity can be a challenge, making AI-powered platforms difficult to deploy or sustain.
Moreover, this economic divide risks widening existing inequalities in the agricultural sector. Large farms equipped with AI can optimize inputs, predict market trends, and increase output, while smaller farms lag behind. This technology gap may contribute to the consolidation of farmland and the marginalization of rural communities already facing systemic disadvantages.
Another underreported issue is dependency. As farmers grow reliant on proprietary AI platforms, they may lose bargaining power and flexibility. Subscription-based models, common in agri-tech, can trap users in expensive ecosystems that benefit vendors more than the farmers themselves.
To truly harness AI’s potential, economic models must be inclusive, scalable, and supportive of all farm sizes, ensuring that innovation doesn’t come at the cost of fairness or long-term sustainability.
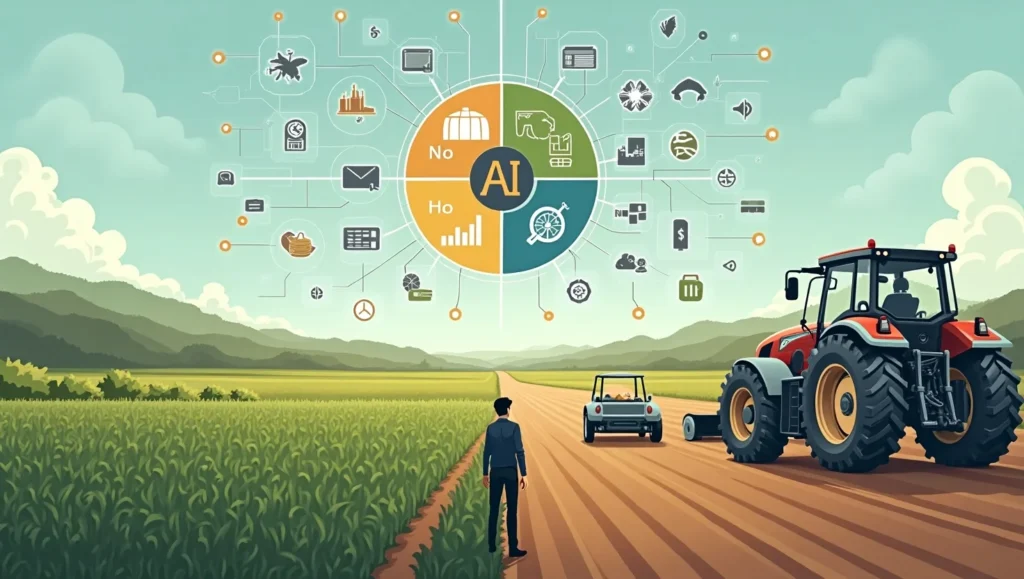
5. Regulatory Landscape and Policy Gaps
As AI technologies reshape agriculture, regulation is struggling to keep pace. Unlike other sectors where AI deployment is governed by well-defined rules, farming lacks robust, standardized policies to guide ethical and economic use. This regulatory vacuum leaves critical issues, like data privacy, liability, and fairness, largely unaddressed, creating uncertainty for farmers, agri-tech developers, and policymakers alike.
Currently, most countries have fragmented or non-existent legislation surrounding AI in agriculture. For example, there are no universal standards defining who owns farm-generated data or how it can be used. Without legal clarity, tech companies often draft terms of service that favor their interests, leaving farmers vulnerable to data exploitation or misuse. This imbalance calls for comprehensive data governance frameworks that prioritize farmer rights and transparency.
Liability is another grey area. When AI systems malfunction or deliver faulty advice, such as misidentifying crop diseases or miscalculating irrigation needs, it’s unclear who is responsible: the farmer, the software provider, or the hardware manufacturer? This lack of accountability discourages adoption and raises ethical concerns.
Additionally, there’s little regulatory focus on ensuring equitable access to AI. Without targeted incentives or subsidies, small-scale farmers may be left behind, deepening rural inequalities. Public policies must bridge this digital divide through education, infrastructure development, and inclusive financing models.
To ensure the ethical and sustainable integration of AI in agriculture, governments must act decisively. Policies need to be proactive, not reactive, encouraging innovation while protecting the interests of those who grow our food.
6. Conclusion: A Path Forward for Ethical and Economic Challenges
The integration of AI into agriculture offers immense potential, but only if it’s guided by ethics, equity, and sound policy. While smart farming solutions can boost productivity, optimize resource use, and help address global food security, they also bring forth a host of challenges that cannot be ignored. From data privacy concerns and biased algorithms to financial exclusion and unclear regulations, the risks are real—and unevenly distributed.
To ensure AI truly benefits all farmers, a multi-stakeholder approach is essential. Policymakers must prioritize the creation of clear legal frameworks that address data ownership, algorithmic transparency, and accountability. Meanwhile, agri-tech developers should adopt ethical design principles, ensuring that their tools are inclusive, accessible, and explainable, even to those without advanced technical knowledge.
Equally important is investment in digital literacy and rural infrastructure. By empowering smallholder farmers with the skills and tools to navigate AI-driven platforms, we can close the technological gap and prevent further consolidation of power in the hands of a few.
AI in agriculture should not be a race toward automation at all costs, it should be a thoughtful evolution that centers farmers, respects their autonomy, and supports sustainable food systems. If we want to cultivate a future where AI genuinely enhances agriculture, we must plant the seeds of fairness, transparency, and shared prosperity today.
FAQs:
Q1. What are the main ethical issues related to AI in agriculture?
AI in agriculture raises concerns about data privacy, biased algorithms, loss of farmer autonomy, and unequal access to technology, especially for smallholder farmers.
Q2. How does AI affect the economic sustainability of farming?
While AI can improve efficiency and yields, high implementation costs and limited digital infrastructure can make adoption economically unfeasible for smaller farms.
Q3. Who owns the data collected by AI tools on farms?
Data ownership remains unclear in many cases. Often, agri-tech companies control the data collected, leaving farmers with limited rights or access to their own information.
Q4. Why is AI adoption in agriculture still low?
Low AI adoption is due to high costs, lack of digital literacy, insufficient internet access, and the absence of clear policies supporting rural and small-scale farmers.
Q5. What policies are needed to regulate AI in agriculture?
Governments need to create transparent policies on data governance, liability, algorithmic fairness, and access to ensure ethical and equitable AI integration in agriculture.
Leave a comment