1. Introduction:
Farming today isn’t what it used to be. As climate change intensifies, traditional agricultural practices struggle to keep up with extreme weather, unpredictable yields, and resource limitations. These challenges aren’t just environmental—they threaten global food security and farmer livelihoods. But there’s a shift happening beneath the soil: machine learning in agriculture is redefining how we grow our food.
Also known as a subset of artificial intelligence, machine learning empowers smart farming by using real-time data and pattern recognition to guide farming decisions. It supports climate-resilient agriculture by helping farmers predict weather impacts, optimize water usage, and reduce crop failure risks.
This isn’t just about big agribusiness. Smallholder farmers, particularly in climate-vulnerable regions, are beginning to adopt AI-based early warning systems and precision farming tools that help them make smarter, more sustainable choices. From pest detection to crop yield forecasting, machine learning provides scalable solutions to age-old problems.
In this article, we’ll explore how machine learning is powering a new generation of AI innovations in agriculture, making farms more efficient, sustainable, and better prepared for a changing climate. You’ll discover the most impactful use cases, real-world examples, and how these technologies can transform farming at every scale.
2. Understanding the Role of Climate-Resilient Agriculture:
At the core of today’s digital transformation in farming is machine learning (ML)—a vital tool in enabling precision agriculture, smart farming, and climate-resilient practices. Unlike traditional methods, which rely heavily on manual observation and guesswork, ML uses massive datasets to uncover patterns and deliver real-time insights.
Here’s how it works: sensors, drones, satellites, and farm equipment collect detailed data on soil health, temperature, rainfall, pest presence, and crop growth. Machine learning algorithms then analyze this data to help farmers decide when to irrigate, fertilize, or harvest—maximizing yield and minimizing waste.
These ML-driven technologies form the backbone of modern agricultural data analytics. For example, smart irrigation systems can automatically adjust watering schedules based on weather predictions, while pest detection models alert farmers before infestations spread. This level of predictive accuracy allows even smallholder farmers to reduce costs and build more climate-smart farming systems.
A common question is: “What kind of data is most useful for machine learning in farming?”
Answer: Diverse, continuous, and context-rich data—including field imagery, soil samples, weather logs, and even equipment usage. The more detailed the dataset, the more effective the machine learning model.
By integrating ML into everyday practices, farmers can future-proof their operations, achieving sustainability, productivity, and resilience in the face of a changing climate.
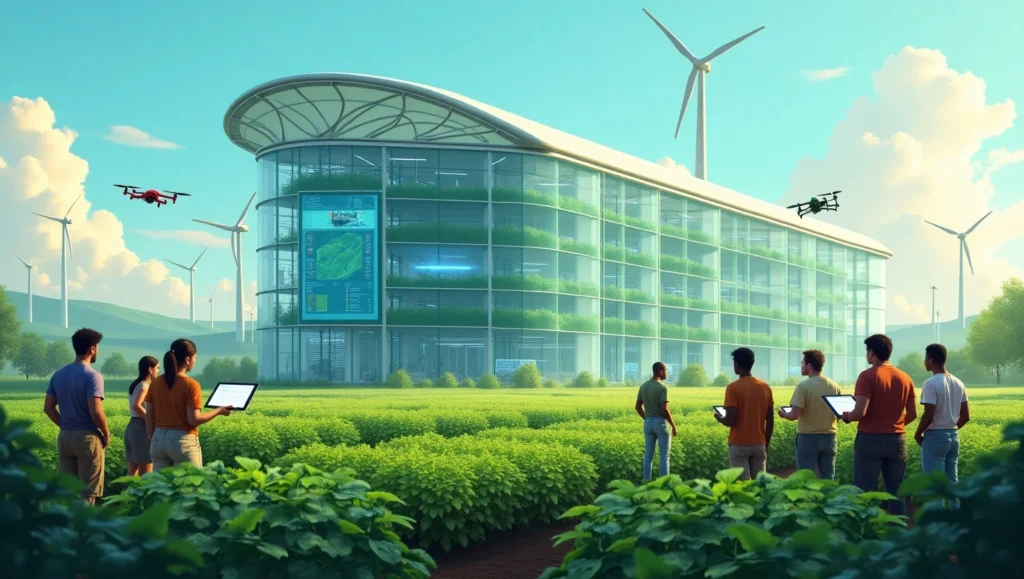
3. Major Use Cases of Machine Learning in Agriculture
Machine learning isn’t just a buzzword in agtech—it’s actively transforming how farms operate day to day. By automating critical decisions and providing deeper insights, machine learning in farming addresses a wide range of real-world challenges.
3.1 Crop Monitoring and Health Diagnosis
ML-powered image recognition tools analyze drone and satellite imagery to detect diseases, nutrient deficiencies, and pest damage—often before the human eye can. This early detection system is vital for preventing crop failure and reducing chemical use.
3.2 Smart Irrigation and Water Management
Water scarcity is a growing concern, but smart agriculture technologies use ML models to calculate optimal watering times and amounts. These systems analyze weather forecasts, soil moisture, and crop type to conserve water without sacrificing yield.
3.3 Pest and Weed Detection
By analyzing patterns in field imagery and sensor data, ML algorithms can detect the early onset of pest infestations or weed growth. This enables precision agriculture by targeting only affected zones with minimal pesticide use.
3.4 Yield Prediction and Forecasting
One of the most powerful applications of predictive farming is yield estimation. ML models factor in weather trends, soil conditions, and plant growth rates to accurately forecast harvest outcomes, critical for both planning and supply chain management.
3.5 Climate Resilience and Risk Assessment
ML helps farmers prepare for weather extremes and seasonal variability by modeling risk and offering adaptive planting schedules, making it a cornerstone of climate-smart agriculture.
4. The Economic and Environmental Impact of Machine Learning in Farming
Machine learning (ML) in agriculture doesn’t just improve crop yields—it also drives economic growth and environmental sustainability. The economic impact is significant, as smart farming technologies enable farmers to reduce costs, optimize resources, and boost productivity.
4.1 Cost Savings and Profitability
By reducing waste in areas like water and fertilizer usage, ML allows farmers to lower input costs. For instance, predictive farming tools that forecast weather conditions can prevent over-application of fertilizers, saving money and reducing environmental runoff. Additionally, improved pest control means fewer chemicals, lowering both costs and environmental damage.
4.2 Sustainability and Resource Efficiency
On the environmental side, ML helps climate-smart farming by minimizing the use of water and synthetic fertilizers, which are both energy-intensive and contribute to greenhouse gas emissions. By using precision agriculture methods, farmers can target inputs precisely, reducing their carbon footprint and conserving valuable resources. These sustainable practices lead to more resilient food systems capable of withstanding the impacts of climate change.
4.3 Boosting Long-Term Productivity
Overall, ML empowers farmers with tools that lead to healthier soils, better crop rotations, and more productive land. Over time, this results in sustainable farming practices that contribute to the broader goal of food security while supporting environmental conservation.
By investing in ML-driven technologies, farmers position themselves for long-term growth, addressing both profitability and sustainability in one sweep.
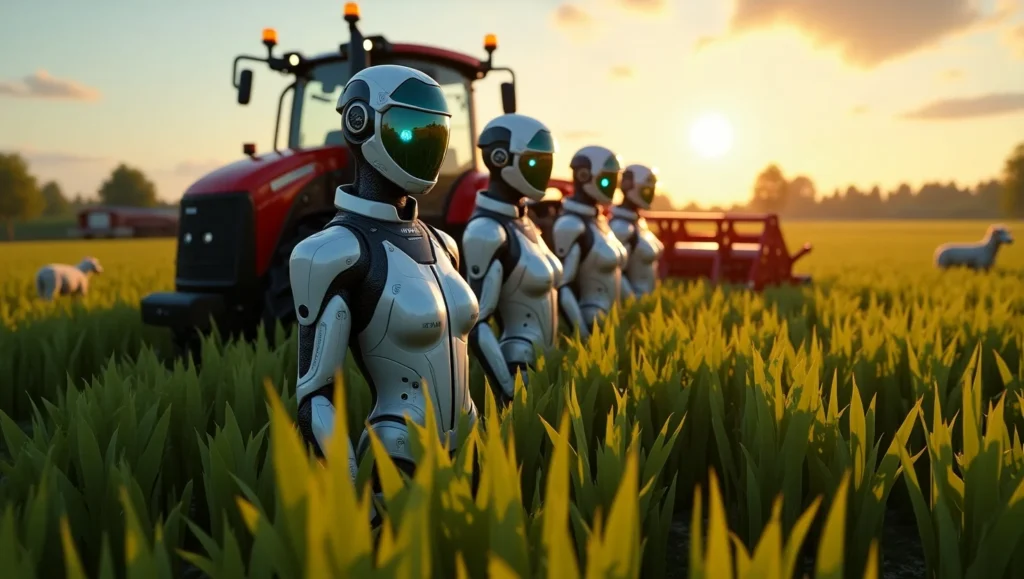
5. Integration with Traditional Farming Practices
It is unlocked when integrated with traditional farming practices. Many farmers, especially in rural or developing areas, rely on time-tested methods passed down through generations. ML doesn’t replace these practices—it enhances them, bringing precision and efficiency to the table.
For instance, farmers can still rely on traditional crop rotation and organic farming techniques while using AI-driven pest detection or soil health monitoring tools to optimize their efforts. By adopting smart farming technologies, farmers can increase their yields, reduce waste, and make more informed decisions, without abandoning the approaches that have worked for them historically.
Incorporating ML may require some initial investment in training or technology, but mobile-friendly platforms and affordable sensors are making these tools more accessible than ever. Moreover, as data accessibility improves, farmers gain better control over their land and resources, allowing them to preserve their legacy while embracing the future.
For many, the key challenge is education: bridging the knowledge gap between traditional farming wisdom and modern technology. By offering hands-on training and easy-to-use tools, ML can be seamlessly integrated into existing agricultural systems, creating a sustainable path forward for farmers worldwide.
6. Future Innovations and AI Collaboration in Agriculture
The future of machine learning in farming looks incredibly promising, with continuous advancements shaping the next generation of agricultural technologies. As AI innovation accelerates, new tools are emerging that further enhance the accuracy and efficiency of precision farming.
6.1 AI and Robotics Integration
Farmers are already beginning to use autonomous tractors, drones, and even robotic harvesters. These systems are powered by ML algorithms that enable machines to perform tasks traditionally done by humans. As AI collaboration expands, farmers can expect even more sophisticated tools, such as self-learning robots capable of identifying and managing complex agricultural tasks.
6.2 Predictive Modeling for Supply Chains
ML will also play a pivotal role in transforming agricultural supply chains. By analyzing real-time data, predictive models can optimize routes, forecast demand, and improve storage conditions, making the entire system more efficient and less wasteful.
6.3 Climate and Sustainability Advances
Looking ahead, machine learning will continue to play a critical role in climate-smart farming initiatives. ML can help farmers predict the impact of climate events, monitor soil health, and adapt crop planning for changing environmental conditions, leading to more sustainable practices.
The future of farming is set to be a collaborative effort between AI, machine learning, and traditional agricultural knowledge, creating a more resilient, efficient, and sustainable food production system.
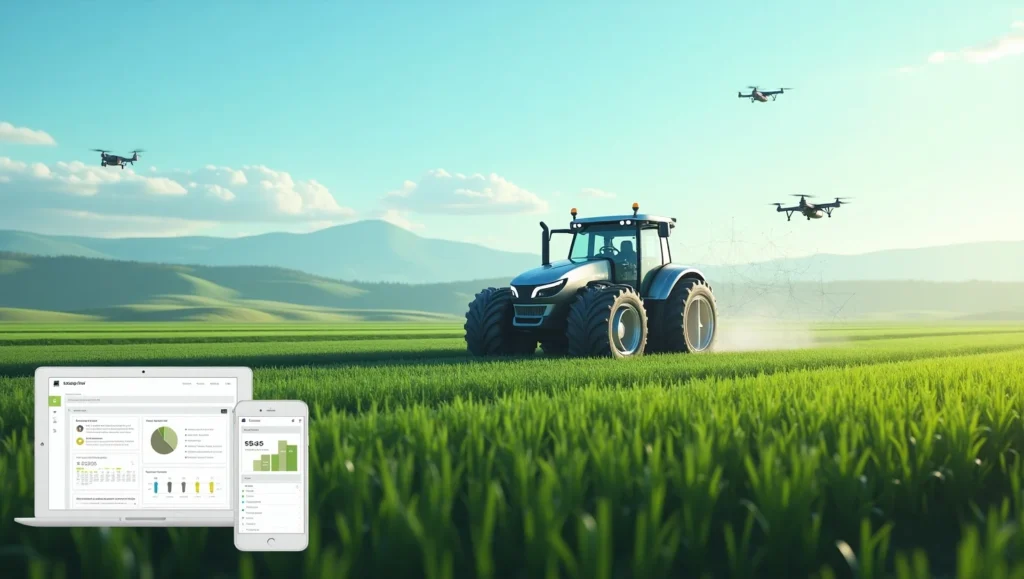
7. Conclusion & Actionable Insights
Machine learning is transforming agriculture, making it smarter, more efficient, and adaptable to the challenges posed by climate change. From precision farming to climate-smart decision-making, ML provides farmers with the tools to optimize resources, boost yields, and create more sustainable food systems.
As the technology advances, it’s essential for farmers to embrace these innovations while integrating them with their traditional practices. Data-driven insights and predictive models will continue to evolve, offering even more opportunities for growth and resilience in farming.
Actionable Steps for Farmers:
- Adopt AI-powered tools like smart irrigation systems and crop health monitors.
- Invest in data collection technologies such as drones or soil sensors for better decision-making.
- Leverage predictive farming models to forecast weather and optimize crop cycles.
- Stay informed about new AI innovations to enhance your farm’s productivity and sustainability.
Machine learning isn’t just the future—it’s the present. By combining technology with tradition, farmers can navigate the evolving agricultural landscape, ensuring productivity and sustainability for generations to come.
FAQs:
1. How does AI assist farmers in adapting to climate change?
AI helps farmers adapt to climate change by analyzing vast datasets—such as weather patterns, soil conditions, and crop health—to provide actionable insights. These insights enable farmers to make informed decisions on irrigation, planting schedules, and resource allocation, thereby enhancing resilience against climate-induced challenges .
2. Can AI help prevent crop failures due to pests and diseases?
Yes, AI can predict and detect pest infestations and crop diseases early by analyzing data from sensors, drones, and satellite imagery. This early detection allows for timely interventions, reducing the risk of widespread crop failures .
3. What role does AI play in improving crop yields?
AI optimizes farming practices by providing precise recommendations on fertilization, irrigation, and harvesting times. By tailoring these practices to specific field conditions, AI contributes to increased crop yields and more efficient resource use .
4. Are there real-world examples of AI helping farmers combat climate challenges?
Yes, companies like SupPlant use AI-driven systems to provide farmers with real-time data on crop and weather conditions, enabling better decision-making. Such technologies have been shown to save water usage by up to 30% and boost crop yields by 5–10% .
5. Is AI accessible to small-scale farmers in developing countries?
Efforts are underway to make AI tools more accessible to smallholder farmers, including mobile applications that provide localized advice. However, challenges such as limited internet connectivity and digital literacy still need to be addressed to ensure widespread adoption .
Leave a comment