1. Introduction
This is where machine learning in farming is beginning to revolutionize the field. By analyzing massive amounts of data, machine learning allows computers to identify patterns, learn from past experiences, and make informed decisions—without needing constant human instructions. It brings the power of automation and precision to the farm.
Across the globe, machine learning applications in agriculture are helping farmers predict crop yields, detect diseases early, optimize irrigation, and even guide autonomous farming equipment. These tools turn raw field data into actionable insights, allowing for smarter, more efficient farming practices.
But machine learning isn’t just about convenience—it’s about survival. As the agricultural industry navigates extreme weather, resource limitations, and increasing food demand, technology must play a central role in the solution.
In this article, we’ll explore the urgent challenges facing agriculture today, dive into how machine learning works in real farming contexts, and showcase real-world success stories. Whether you’re a farmer, researcher, or tech enthusiast, this journey into smarter agriculture will show you how innovation is sowing the seeds for a more sustainable future.
2. The Agricultural Crisis: Why Smarter Solutions Are Needed
Agriculture, the backbone of human civilization, is under increasing strain. As the global population heads toward 10 billion by 2050, the pressure to produce more food with fewer resources is mounting. Traditional farming methods—while dependable in the past—are now struggling to keep up with the growing demand, climate unpredictability, and ecological sustainability.
One of the most pressing food production challenges is resource limitation. Farmers are faced with shrinking arable land, water scarcity, and soil degradation. Add to that the unpredictability of weather patterns and increasing incidences of pests and diseases, and it’s clear that conventional approaches simply aren’t enough anymore.
Moreover, many small to medium-sized farms operate on thin margins and lack access to the latest technologies or scientific guidance. As a result, decisions are often based on gut instinct rather than data. This leads to inefficiencies, wasted inputs like water or fertilizer, and missed opportunities for maximizing yield.
That’s where smarter agriculture comes into play. By introducing digital technologies—particularly machine learning—farmers can now analyze data from their fields in real time, detect issues before they escalate, and predict crop performance more accurately. These tools are helping farms become not just more productive but also more sustainable.
Machine learning enables precision. Whether it’s determining the optimal time for irrigation, identifying nutrient deficiencies, or forecasting pest outbreaks, intelligent algorithms empower farmers to act with confidence and foresight.
In a world where every drop of water and every seed counts, adopting smarter agriculture isn’t just an option—it’s becoming a necessity. The future of food security depends on our ability to farm efficiently, sustainably, and intelligently.
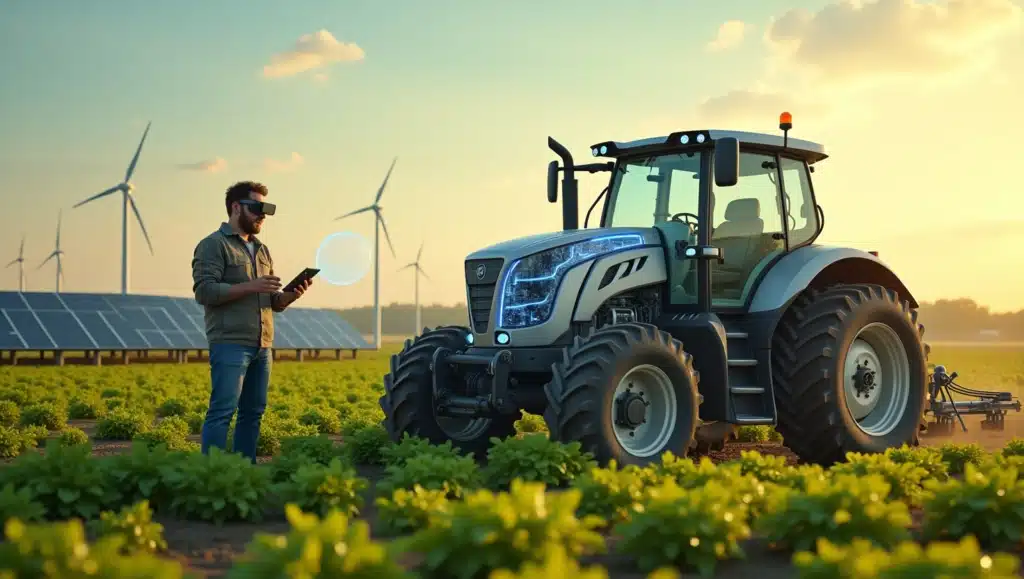
3. What Makes Machine Learning ‘Smart’ for Farming?
To understand why machine learning is making waves in modern farming, we first need to explore what makes it “smart.” Unlike traditional software that follows fixed rules, machine learning systems continuously improve by learning from data—just like a farmer gains experience over the years by observing crops and outcomes.
In the context of precision agriculture, this means using data from satellites, drones, sensors, and farm equipment to make highly accurate decisions. Every field, crop, and even plant can be monitored in real time. Soil conditions, weather patterns, water usage, and pest presence are all turned into measurable data points. Machine learning algorithms process this data to find trends and predict outcomes faster and more precisely than any human could.
One of the most powerful uses of this technology is machine learning for crop prediction. By analyzing historical weather, soil data, and past yields, ML models can forecast harvest outcomes weeks or even months in advance. This empowers farmers to plan better, choosing the right crops, optimizing fertilization schedules, and managing resources more effectively.
Moreover, these smart systems aren’t one-size-fits-all. They adapt to specific farm conditions. A machine learning model trained on a vineyard in California might look very different from one used in rice paddies in Southeast Asia.
Machine learning is turning agriculture from reactive to proactive. Instead of responding to problems after they occur, farmers can now anticipate them and take preventive action. This shift from intuition to information is what truly defines smart farming—and it’s just the beginning.
4. Real-World Examples of Machine Learning in Use
While the concept of machine learning might sound complex or futuristic, it’s already being used on farms around the world to solve real problems. These practical examples show how smart farming is no longer a buzzword—it’s a working reality.
Case Study 1: Smart Irrigation in California
In the drought-prone Central Valley, farmers are using AI-powered systems to monitor soil moisture and weather forecasts. These systems automatically determine when and how much water is needed, helping reduce waste and improve crop quality. This is smart farming in action—technology making decisions in real time, based on real data.
Case Study 2: Pest and Disease Prediction in India
Farmers in India are using mobile-based machine learning platforms that analyze images of crops to detect early signs of pest infestations and diseases. These tools are trained using thousands of labeled images, allowing the system to flag problems before they become widespread.
Case Study 3: Vineyard Management in France
Using drones and sensors, vineyards in France are gathering data on leaf color, soil condition, and grape development. The data is then processed by machine learning algorithms to predict optimal harvest times and guide decisions on pruning or fertilization. These are advanced AI applications in crop management that are boosting both efficiency and quality.
Each of these examples shows how machine learning is providing solutions tailored to different types of farming. From conserving water to maximizing yield, smart farming tools are helping growers make better decisions, reduce risks, and increase profits.
These success stories are proof that the future of agriculture isn’t waiting—it’s already growing.
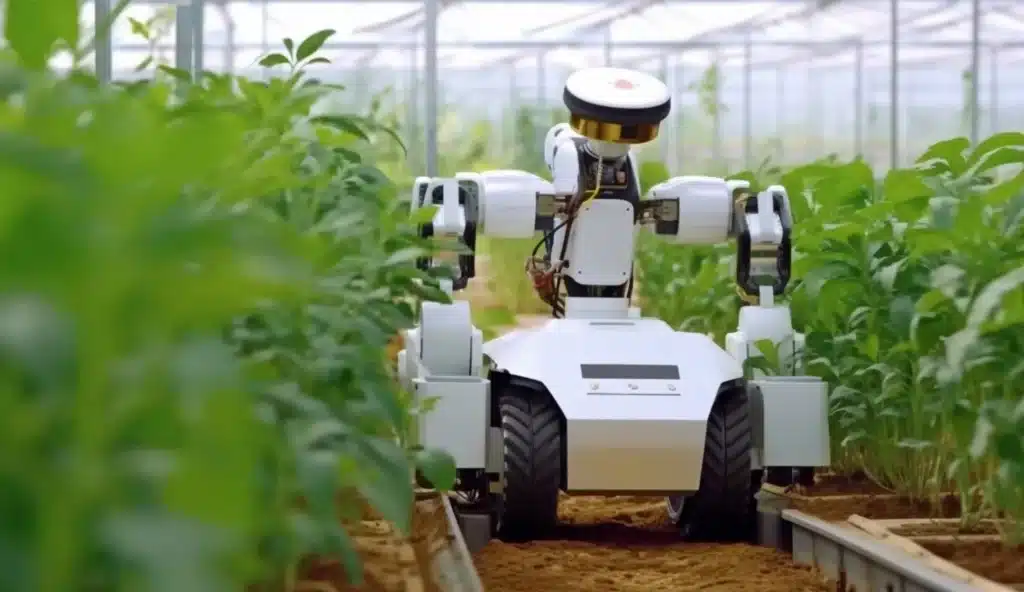
5. How Machine Learning Enhances Farm Operations
As agriculture embraces the digital age, the benefits of AI in agriculture are becoming more visible across day-to-day farm operations. From planning and planting to harvesting and storage, machine learning is optimizing every step of the farming process—bringing in a new era of efficiency.
Imagine a farm that automatically adjusts fertilizer usage based on real-time soil data or a system that detects crop stress before it’s visible to the human eye. These aren’t futuristic concepts—they’re happening now, thanks to AI-driven farming solutions.
5.1 Efficiency at Scale
Machine learning helps reduce guesswork. For instance, AI tools can analyze data from previous harvests to suggest the best planting schedules, the right seed varieties for local soil conditions, and even when to apply pesticides to minimize loss. This precision not only saves money but also improves productivity.
5.2 Resource Optimization
Smart algorithms manage water, fertilizer, and energy more precisely than manual methods. This means less waste, lower costs, and a more sustainable approach to farming.
5.3 Data-Driven Decisions
Rather than relying solely on experience or tradition, farmers now have access to actionable insights. AI platforms offer dashboards and alerts that simplify complex data, making it easier for farmers to make the right choices quickly.
Whether it’s smallholder farmers using mobile apps or large agribusinesses deploying drone analytics, AI-driven farming solutions are reshaping how work gets done on the field.
The result? Farms that are more productive, sustainable, and resilient—built on a foundation of smart data and smarter tools.
6. Barriers to Adoption & How to Overcome Them
While the potential of AI and farm automation is exciting, the path to widespread adoption is not without hurdles. Many farmers, especially those in developing regions or operating on tight budgets, face real challenges in implementing machine learning tools on their farms.
6.1 High Initial Costs
One of the biggest machine learning adoption challenges is the upfront investment. Purchasing sensors, drones, and data platforms can be expensive—especially for small or family-run farms. Although these technologies pay off in the long term, the initial costs often prevent farmers from taking the first step.
6.2 Lack of Technical Skills
Operating AI systems requires a basic understanding of data and digital tools. Many farmers may not have the time or training to analyze dashboards or interpret machine learning models. This gap limits the full use of smart technologies, even when they’re available.
6.3 Connectivity and Infrastructure
Reliable internet access is essential for real-time data processing and cloud-based tools. Rural areas, however, often struggle with poor connectivity, making it hard to implement advanced farm automation solutions.
6.4 Overcoming These Challenges
Thankfully, solutions are emerging. Governments and private companies are offering subsidized AI tools and educational programs. Some platforms are now mobile-based and user-friendly, requiring minimal tech expertise. Others use edge computing—processing data directly on the farm—to reduce dependence on the internet.
Collaborative efforts among agri-tech startups, NGOs, and policymakers are also making smart farming more inclusive and accessible.
Overcoming these barriers is crucial. Because once the gap is bridged, machine learning doesn’t just help individual farms—it lifts entire agricultural communities into the future.
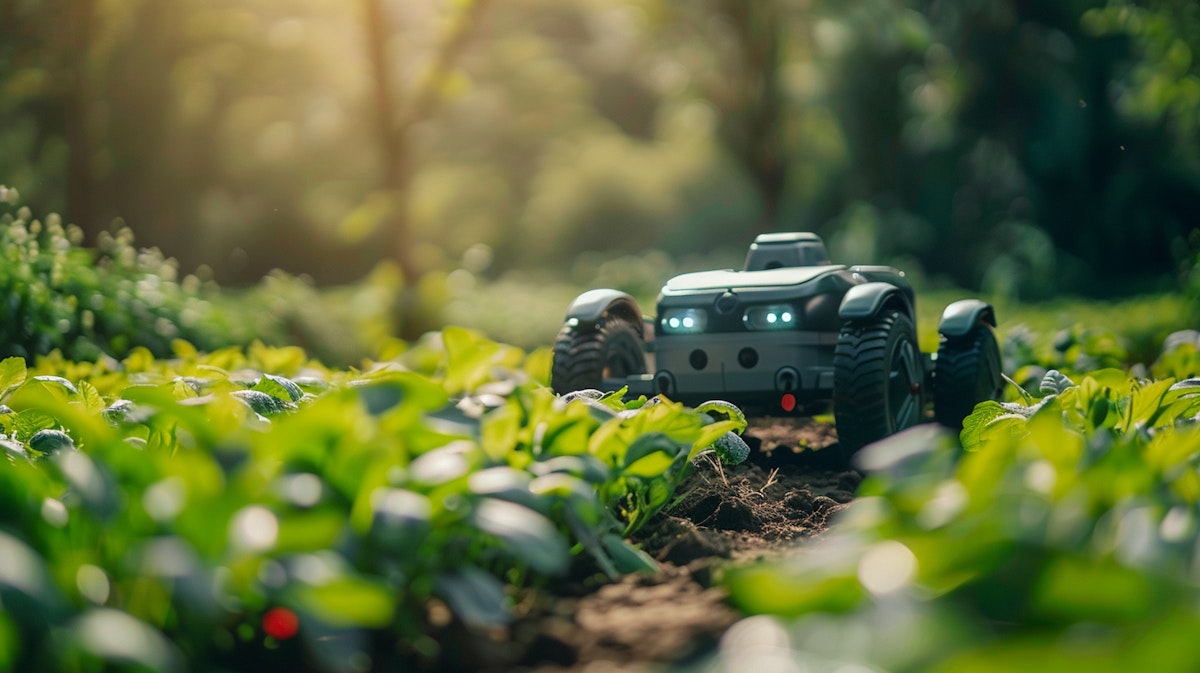
7. Getting Started with Machine Learning on Your Farm
Taking the first step into AI-powered farming might feel overwhelming, but it doesn’t have to be. Thanks to advances in agricultural data analytics, machine learning is becoming more accessible—even for smaller farms with limited resources.
The first thing to understand is that you don’t need to go all-in at once. Start small. Many farms begin by integrating simple data-collection tools like soil moisture sensors, weather stations, or drone imagery. These tools provide the raw data that powers intelligent decision-making.
Once you’ve started gathering data, you can explore agricultural machine learning tools to help you make sense of it. Platforms like CropX, FarmLogs, or Taranis offer analytics dashboards that break down complex patterns into easy-to-follow insights. Some solutions even come with mobile apps, allowing farmers to track crop health or receive irrigation alerts directly from their phones.
For livestock farmers, tools like Connecterra use machine learning to monitor animal health and predict behavioral changes, reducing disease risk and improving milk or meat output.
Don’t have tech experience? No problem. Many solutions now come with intuitive interfaces and require minimal training. Some agricultural extension services also offer hands-on support to help you get started.
The key is to begin with a problem you want to solve—maybe it’s reducing water use, improving pest control, or increasing yield—and then explore which tools fit your needs and budget.
With the right combination of data and technology, even a small step toward agricultural data analytics can lead to big improvements in productivity, sustainability, and profitability.
8. The Road Ahead: What’s Next for Machine Learning in Agriculture
As machine learning continues to evolve, it’s not just transforming farming today—it’s laying the foundation for tomorrow’s food systems. The future of agriculture is not only digital; it’s intelligent, autonomous, and deeply connected.
One of the most exciting advancements is the rise of agricultural robotics. These are autonomous machines—like AI-powered harvesters, robotic weeders, and drone-based crop monitors—that use machine learning to operate independently. They can work around the clock, reduce labor costs, and make ultra-precise decisions that improve both yield and sustainability.
Imagine a robot that identifies weeds with computer vision and removes them individually, without harming nearby crops or relying on chemical sprays. That’s not science fiction—it’s happening on test farms right now.
But robotics is just the beginning. The integration of next-gen agriculture technologies such as blockchain for supply chain transparency, edge computing for real-time data processing, and synthetic sensors for nutrient detection are rapidly changing the way we think about farming.
The convergence of technologies—AI, IoT, robotics, and big data—is creating a fully digital ecosystem where farms are more efficient, sustainable, and resilient.
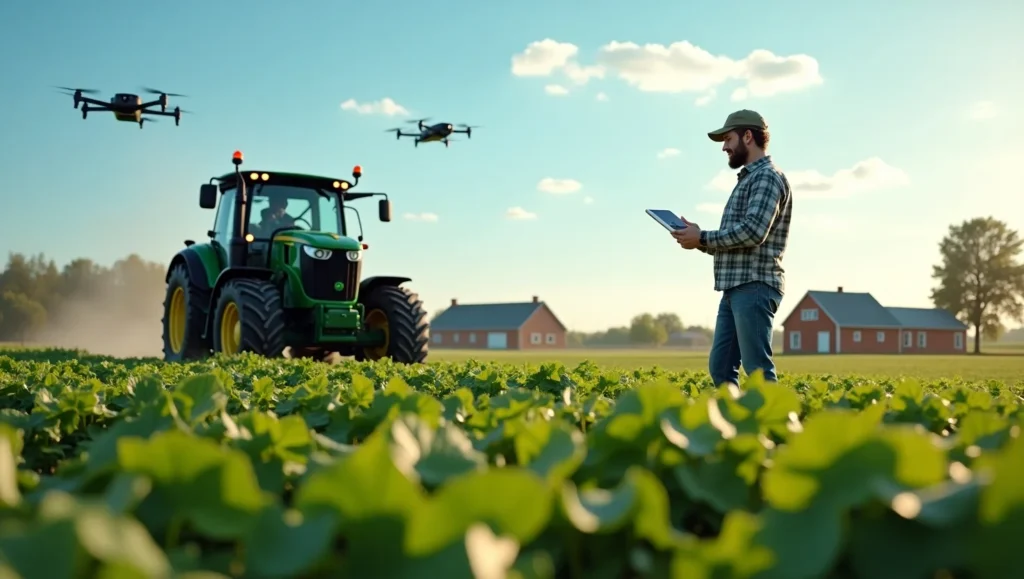
9. Conclusion & Call to Action
The age of data-driven farming is here, and its impact is undeniable. From planting the first seed to delivering food to markets, machine learning is helping farmers work smarter—not harder. The combination of AI, data, and real-time analytics is reshaping every step of the agricultural journey.
Technologies like IoT in agriculture have already made it easier to gather data from the field—whether it’s soil moisture, temperature, or crop growth metrics. But what makes this data truly powerful is how it’s processed and applied. That’s where machine learning shines.
Through AI-powered crop monitoring, farmers can now detect diseases before they spread, apply the right treatment at the right time, and forecast yields with a level of accuracy that was once impossible. These tools are not replacing farmers—they’re empowering them to make faster, more confident, and more sustainable decisions.
But as we’ve seen throughout this article, adopting machine learning in farming comes with challenges. High costs, technical barriers, and infrastructure gaps still stand in the way. The good news? Solutions are emerging, support systems are growing, and more farmers are beginning to see the value of this transformative technology.
If you’re a farmer, researcher, or agribusiness leader, now is the time to act. Start exploring AI tools, pilot small projects, and embrace the shift toward smarter agriculture. Innovation is no longer optional—it’s essential.
Ready to take the first step?
Explore beginner-friendly machine learning platforms, connect with local agri-tech networks, and stay informed about the latest trends in smart farming.
Because the future of agriculture isn’t just growing—it’s learning, adapting, and evolving with every harvest.
FAQs:
Q1. How is machine learning used in agriculture?
Machine learning helps in predicting crop yields, detecting diseases, and optimizing irrigation in modern farming.
Q2. What are the benefits of machine learning in farming?
It increases productivity, reduces costs, and improves decision-making through data-driven insights.
Q3. Can machine learning improve crop management?
Yes, machine learning enables precise monitoring of soil, weather, and plant health for better crop outcomes.
Q4. What are some real-world examples of ML in agriculture?
Companies like John Deere and IBM use ML for autonomous tractors, pest prediction, and smart irrigation systems.
Q5. Is machine learning cost-effective for small farms?
While initial costs can be high, many affordable ML-based tools are emerging for small and mid-sized farms.
Leave a comment